LANDFIRE Data and Applications
Links
- Document: Report (14.6 MB pdf) , HTML , XML
- Download citation as: RIS | Dublin Core
Abstract
Wildfire risk, species conservation, and ecosystem management all depend on seamless spatial data. LANDFIRE may already be supporting your mission if you have ever asked questions like these:
-
What is the wildfire risk within a particular landscape?
-
Where can I get data to evaluate fauna habitats?
-
If ignited, how might a wildfire move through a particular landscape?
-
How does the vegetation cover in one area compare with the vegetation in another area?
-
How have disturbances in the past affected current forest conditions?
-
What is the spatial distribution of a certain vegetation type?
-
Where can I find spatial vegetation and structure data for all lands, regardless of ownership?
National LANDFIRE datasets can help answer all these questions for areas of interest within the United States and insular areas at the 30-meter pixel level. LANDFIRE is a Federal program that provides a suite of spatial datasets indicating areas of disturbance, vegetation and fuels distributions and structure, and historical conditions. Although LANDFIRE is the definitive dataset used by the interagency fire community for surface and canopy fuels, the program also maps more than 30 spatial datasets that can be used for a variety of purposes.
Mapping Process
LANDFIRE assigns disturbance, vegetation, fuel, and fire regime classifications to every pixel within every 30 meters of land in the United States. A good example of these methods is the process of assigning a pixel to an ecological system or to a U.S. National Vegetation Classification group, LANDFIRE’s two vegetation type classifications. LANDFIRE uses the relation between satellite image surface reflectance and field plots to extrapolate vegetation classes to other pixels containing similar information. The satellite reflectance data come primarily from 30-meter Landsat sensors and provide a consistent dataset that has been the basis for LANDFIRE mapping since 2004.
LANDFIRE Data—Essential for National Fire Assessments and Managing Large Wildfires
LANDFIRE data are foundational for wildfire hazard and risk studies. Firesheds, potential operational delineations (commonly known as PODs), wildfire risk assessments (fig. 1), and other national efforts rely on LANDFIRE data to power fire behavior models. Fire Simulation (known as FSim), a frequently used landscape-level fire behavior model, requires LANDFIRE fuel models, canopy information, and topography inputs to begin model runs.
Most large wildfires have a Federal interagency incident command that accesses the Wildland Fire Decision Support System (WFDSS). As part of its capabilities, the WFDSS encompasses several spatial fire behavior models such as Basic Fire Behavior, Near-Term Fire Behavior, and Fire Spread Probability (more commonly known as FSPro). All WFDSS spatial models require LANDFIRE fuel and topography layers as inputs.
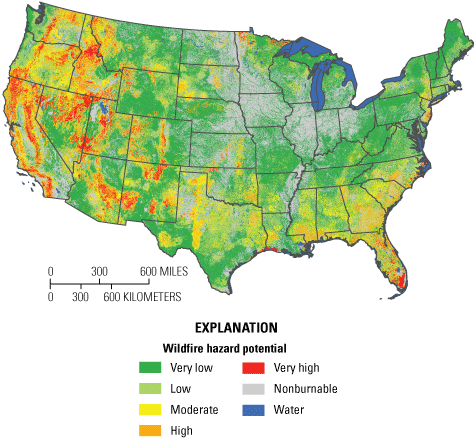
Wildfire hazard based on LANDFIRE 2014 fuels and topography.
LANDFIRE Data Inform Habitat Research
LANDFIRE data also have been used for habitat analyses. Here are a few recent examples:
-
Mammals—LANDFIRE canopy attributes were used in habitat preference modeling for Pekania pennanti (fishers; fig. 2) in the northern Rocky Mountains (Krohner and others, 2022).
-
Pollinators—LANDFIRE’s Existing Vegetation Type lifeform feature was used to investigate pollinator habitat restoration potential at solar facilities (Walston and others, 2021).
-
Avian—LANDFIRE’s Existing Vegetation Cover feature was used to investigate avian species richness in pyrodiverse Pinus palustris Mill. (longleaf pine; fig. 3) habitats in Florida (Jorge and others, 2022).
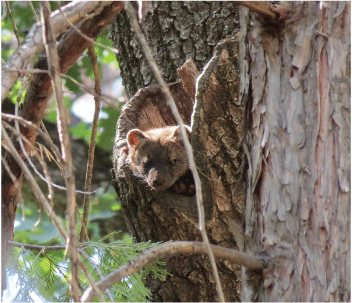
Fisher spotted in the canopy on a national forest in California. Photograph by Zane Miller, Forest Service.
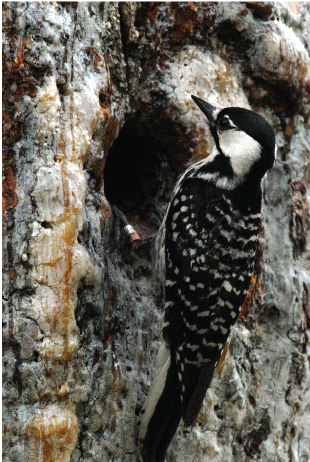
This endangered Leuconotopicus borealis (red-cockaded woodpecker) is feeding its young at the nest in a cavity of a longleaf pine in Georgia (the same ecosystem type studied by Jorge and others [2022]). Active management and restoration of longleaf forests can help recover this species. Photograph by John Maxwell, U.S. Fish and Wildlife Service.
LANDFIRE—High-Quality Annual Disturbance Maps at the Ready
LANDFIRE annual disturbance mapping (fig. 4) has historically been a “means to an end” to help map vegetation and fuel changes caused by disturbance; however, LANDFIRE’s stand-alone annual disturbance layers ranging from 1999 to 2020 are a rich data source for disturbance history data. Where else can you find detailed disturbance information with the source, cause, severity, and detailed notes on how that information was derived?
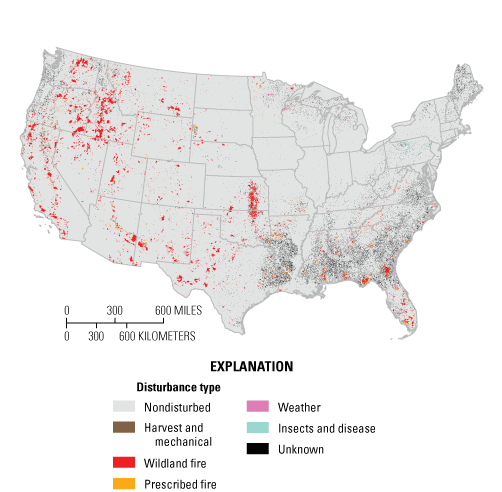
Historical disturbance tracking for 10 years of disturbance types (2006–16).
LANDFIRE Also Has Fire and Treatment Perimeter Data
LANDFIRE’s Events Geodatabase tracks more than 20 years of vegetation disturbance and treatment data. The scientists supporting LANDFIRE have cultivated relationships with local, State, and Federal agencies, along with nonprofit agencies across the Nation, to provide perimeters of change. These perimeters are used to help create our annual disturbance layers and assign disturbance causes such as mechanical, insect and disease, prescribed fire, wildfire, and weather events. Examples of well-known national data sources included are the Forest Service Activity Tracking System (known as FACTS), the National Fire Plan Operations and Reporting System (known as NFPORS), and the National Interagency Fire Center (known as NIFC).
LANDFIRE’s Plot Data and Machine Learning—Keeping Pace
LANDFIRE uses plot data to train our machine learning models, which in turn, create high-quality data and products. In the age of artificial intelligence, the demands for ground-referenced datasets are increasing. Plot data in the LANDFIRE Reference Database originate from a wide range of sources (table 1) across many years. Our LANDFIRE reference team is always searching for plot data to add to the database, so if you know of a plot dataset that applies to vegetation and structure, LANDFIRE probably has it, and if not, we want it!
Table 1.
Primary national, State, and Federal LANDFIRE Reference Database plot dataset contributors.Biophysical Settings and Fire Regimes—A Glimpse into the Past
Biophysical settings (BPS) are useful for anyone trying to understand what the landscape might have looked like before Europeans colonized the continent. By incorporating the fire regimes that most likely existed at that time, BPS indicates the most probable succession state that would have been found at any location. LANDFIRE’s Vegetation Departure (VDep) layer gives an estimate of the difference between historical BPS and current LANDFIRE existing vegetation. This assessment of “departure” from the past may help prioritize land management restoration decisions.
LANDFIRE—Your Source for Disturbance, Vegetation, and Fuel Spatial Data
LANDFIRE is a complete, all-lands program run by the Department of the Interior and the Forest Service and operated by the U.S. Geological Survey. The Nature Conservancy partners with LANDFIRE to help answer application questions, manage informational programs, and model ecosystem dynamics. We urge you to explore LANDFIRE spatial data at https://www.landfire.gov if you need disturbance, vegetation, or fuels information. Contact the LANDFIRE help desk with any questions (helpdesk@landfire.gov).
References Cited
Jorge, M.H., Conner, L.M., Garrison, E.P., and Cherry, M.J., 2022, Avian species richness in a frequently burned ecosystem—A link between pyrodiversity and biodiversity: Landscape Ecology, v. 37, no. 4, p. 983–995. [Also available at https://doi.org/10.1007/s10980-022-01399-8.]
Krohner, J.M., Lukacs, P.M., Inman, R., Sauder, J.D., Gude, J.A., Mosby, C., Coltrane, J.A., Mowry, R.A., and Millspaugh, J.J., 2022, Finding fishers—Determining fisher occupancy in the Northern Rocky Mountains: The Journal of Wildlife Management, v. 86, no. 2. art. e22162, 20 p., accessed March 30, 2022, at https://doi.org/10.1002/jwmg.22162.
Walston, L.J., Li, Y., Hartmann, H.M., Macknick, J., Hanson, A., Nootenboom, C., Lonsdorf, E., and Hellmann, J., 2021, Modeling the ecosystem services of native vegetation management practices at solar energy facilities in the Midwestern United States: Ecosystem Services, v. 47, p. 101–227. [Also available at https://doi.org/10.1016/j.ecoser.2020.101227.]
For more information about this publication, contact:
LANDFIRE help desk
USGS Earth Resources Observation and Science Center
47914 252nd Street
Sioux Falls, SD 57198
605–594–2839
Email: helpdesk@landfire.gov
Publishing support provided by the
Rolla and Sacramento Publishing Service Centers
Suggested Citation
La Puma, I.P., and Hatten, T.D., 2022, LANDFIRE data and applications: U.S. Geological Survey Fact Sheet 2022–3034, 4 p., https://doi.org/10.3133/fs20223034.
ISSN: 2327-6932 (online)
ISSN: 2327-6916 (print)
Study Area
Publication type | Report |
---|---|
Publication Subtype | USGS Numbered Series |
Title | LANDFIRE data and applications |
Series title | Fact Sheet |
Series number | 2022-3034 |
DOI | 10.3133/fs20223034 |
Year Published | 2022 |
Language | English |
Publisher | U.S. Geological Survey |
Publisher location | Reston, VA |
Contributing office(s) | Earth Resources Observation and Science (EROS) Center |
Description | 4 p. |
Country | United States |
Online Only (Y/N) | N |
Google Analytic Metrics | Metrics page |